Processing-in-Memory
Processing deep learning workloads inside memory (메모리 내에서 딥러닝 워크로드 처리)
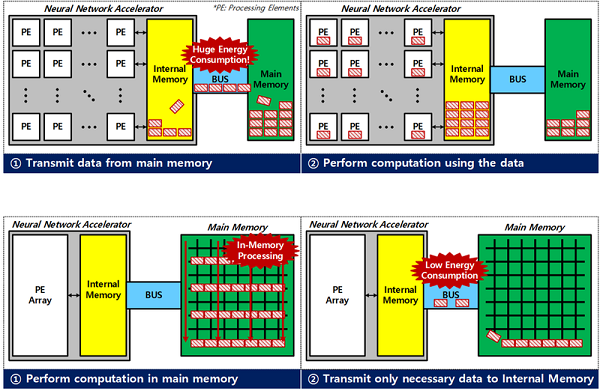
Research Description
Processing-in-memory (PIM) is an innovative computing paradigm that aims to overcome the limitations of the traditional von Neumann architecture, which separates the processing units (CPU/GPU) from the memory units (RAM). This separation creates a bottleneck known as the “memory wall,” where the time and energy costs of data movement between the processor and memory significantly impact overall system performance.
PIM integrates computational capabilities directly into memory units. Instead of transferring data back and forth between the CPU/GPU and RAM, PIM allows for data processing to occur within the memory itself. This approach can drastically reduce data movement, leading to improvements in speed, energy efficiency, and overall system performance.
메모리 내 연산 (Processing-in-memory, PIM)은 전통적인 폰 노이만 아키텍처의 한계를 극복하기 위한 혁신적인 컴퓨팅 패러다임입니다. 폰 노이만 아키텍처에서는 처리 장치 (CPU/GPU)와 메모리 (RAM)가 분리되어 있으며, 이로 인해 “메모리 벽”이라고 불리는 병목 현상이 발생합니다. 이 병목 현상은 프로세서와 메모리 간의 데이터 이동에 드는 시간과 에너지 비용이 전체 시스템 성능에 크게 영향을 미치는 문제를 일으킵니다.
PIM은 연산 능력을 메모리 장치에 직접 통합합니다. CPU/GPU와 RAM 사이에서 데이터를 주고받는 대신, PIM은 메모리 내에서 직접 데이터를 처리할 수 있게 합니다. 이러한 접근 방식은 데이터 이동을 크게 줄여 속도, 에너지 효율성, 전체 시스템 성능을 향상시키는 데 기여할 수 있습니다.
Your Job
- Understanding the concept of computational intensity, memory bandwidth, and memory-bounded workload.
- Understanding various memory technologies.
- Evaluating workloads and identifying computational or memory bottleneck.
-
Designing a novel PIM architecture or PIM software stack.
- 계산 집약도, 메모리 대역폭, 메모리 바운드 워크로드에 대한 이해.
- 다양한 메모리 기술에 대한 이해.
- 워크로드를 평가하고 계산 또는 메모리 병목 현상을 식별.
- 새로운 PIM 아키텍처 또는 PIM 소프트웨어 스택 설계.
Related Papers:
-
SCIESpDRAM: Efficient In-DRAM Acceleration of Sparse Matrix-Vector MultiplicationIEEE Access, vol.12, pp.176009-176021, 2024
-
SCIES-FLASH: A NAND Flash-Based Deep Neural Network Accelerator Exploiting Bit-Level SparsityIEEE Transactions on Computers, vol.71, num.6, pp.1291–1304, 2021
-
Top-TierA PVT-Robust Customized 4T Embedded DRAM Cell Array for Accelerating Binary Neural NetworksIn 2019 IEEE/ACM International Conference on Computer-Aided Design (ICCAD)
-
Top-TierAn Energy-Efficient Processing-in-Memory Architecture for Long Short Term Memory in Spin Orbit Torque MRAMIn 2019 IEEE/ACM International Conference on Computer-Aided Design (ICCAD)
-
Top-TierNAND-Net: Minimizing Computational Complexity of In-Memory Processing for Binary Neural NetworksIn 2019 IEEE International Symposium on High Performance Computer Architecture (HPCA)
-
Top-TierNID: Processing Binary Convolutional Neural Network in Commodity DRAMIn 2018 IEEE/ACM International Conference on Computer-Aided Design (ICCAD)