Efficient AI
Quantization, pruning, and knowledge distillation (AI 모델 경량화를 위한 양자화, 가지치기, 지식 증류)
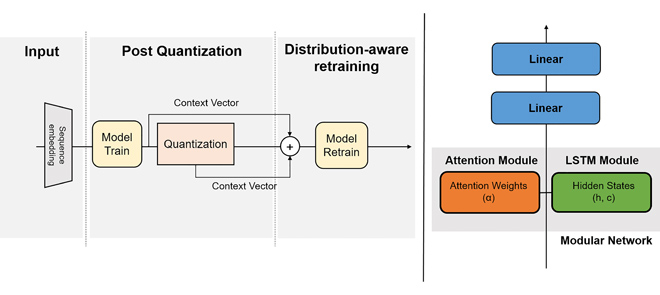
Research Description
Quantization is a technique used to reduce the precision of the numbers used to represent a model’s weights or activations. In neural networks, weights and activations are typically stored as 32-bit floating-point numbers. Quantization reduces this precision to 16-bit, 8-bit, or even lower, thereby reducing the model’s size and increasing its inference speed. We are also looking for an opportunity to make quantization hardware-friendly as many existing quantization methods require specialization in hardware resource.
양자화는 모델의 가중치나 활성화 값을 표현하는 숫자의 정밀도를 줄이는 기술입니다. 신경망에서 가중치와 활성화 값은 일반적으로 32비트 부동소수점 숫자로 저장됩니다. 양자화는 이 정밀도를 16비트, 8비트, 또는 그 이하로 줄여 모델의 크기를 줄이고 추론 속도를 높입니다. 또한, 많은 기존 양자화 방법이 하드웨어 리소스의 특수화를 요구하기 때문에, 우리는 양자화를 하드웨어 친화적으로 만드는 기회를 모색하고 있습니다.
Pruning involves removing less important or redundant weights from a neural network. The goal is to reduce the size of the model and improve its efficiency without significantly sacrificing accuracy. Pruning helps in reducing the model’s computational requirements and memory footprint, making it faster and more efficient. Structured pruning is our special interest as it exploits underlying hardware architecture to improve pruning efficacy.
가지치기는 신경망에서 덜 중요한 또는 중복된 가중치를 제거하는 것을 말합니다. 이 방법의 목표는 정확도를 크게 희생하지 않으면서 모델의 크기를 줄이고 효율성을 높이는 것입니다. 가지치기는 모델의 계산 요구사항과 메모리 사용량을 줄여 더 빠르고 효율적으로 만드는 데 기여합니다. 구조적 가지치기는 하드웨어 아키텍처를 활용하여 가지치기의 효율성을 높이기 때문에 우리가 특히 관심을 가지고 있는 분야입니다.
Knowledge distillation is a process where a smaller, simpler model (student model) is trained to mimic the behavior of a larger, more complex model (teacher model). This technique allows the student model to achieve performance close to that of the teacher model while being much more compact and efficient, making it suitable for deployment in scenarios with limited computational resources.
지식 증류는 더 크고 복잡한 모델 (교사 모델)의 동작을 모방하도록 더 작고 간단한 모델 (학생 모델)을 훈련시키는 과정입니다. 이 기술을 통해 학생 모델은 교사 모델에 가까운 성능을 유지하면서도 훨씬 더 컴팩트하고 효율적으로 설계되며, 제한된 컴퓨팅 리소스가 있는 환경에서 배포하기에 적합합니다.
Your Job
- Understanding of the above concepts.
- Understanding of interaction between these methods and computing hardware.
-
Devising a hardware-friendly methodology.
- 위 개념들에 대한 이해.
- 이러한 방법들과 컴퓨팅 하드웨어 간의 상호작용에 대한 이해.
- 하드웨어 친화적인 방법론 개발.
Related Papers:
-
SCIEPRISM-Med: Parameter-efficient Robust Interdomain Specialty Model for Medical Language TasksIEEE Access, vol.13, pp.4957-4965, 2025Collaborative research conducted with NVIDIA
-
OCW: Enhancing Few-Shot Learning with Optimized Class-Weighting MethodsIn 2024 International Conference on Communications, Computing, Cybersecurity, and Informatics (CCCI)
-
SCIEQ-LAtte: An Efficient and Versatile LSTM Model for Quantized Attention-Based Time Series Forecasting in Building Energy ApplicationsIEEE Access, vol.12, pp.69325-69341, 2024
-
Top-TiereSRCNN: A Framework for Optimizing Super-Resolution Tasks on Diverse Embedded CNN AcceleratorsIn 2019 IEEE/ACM International Conference on Computer-Aided Design (ICCAD)